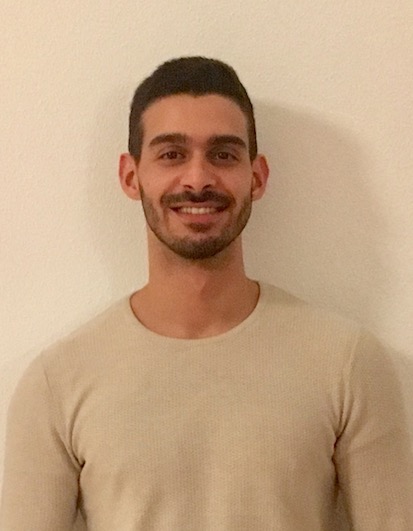
Emanuele Boattini MSc
Leonard S. Ornstein Laboratory, room 0.14
Princetonplein 1, 3584 CC Utrecht
P.O. Box 80 000, 3508 TA Utrecht
The Netherlands
phone: +31 (0)30 253 2831
secretariat: +31 (0)30 253 2952
e-mail: e.boattini@uu.nl
Research
Supervisor: Dr. Laura Filion
Promotor: Prof. dr. ir. Marjolein Dijkstra
Funding: NWO
Employed: 1 September 2017 – 31 August 2021
Neural network based order parameters for classification of binary crystal structures
In many types of research, such as study of crystallization from the undercooled liquid, one needs to be able to distinguish particles that are part of the crystal from those that belong to the liquid. Moreover, it is very often desirable to be able to discriminate between different crystal structures. Ideally, such an assignment should be based on the local environment of the particles only.
Several methods to extract local structural information on a single particle basis have been proposed. Given their success for single component systems, in this study we use the averaged local bond order parameters proposed by Lechner and Dellago [1]. Combinations of such bond order parameters are usually used in pairs in order to identify regions on a plane spanned by the pairs that correspond to different environments – and sometime more than one set of pairs are necessary. While specific combinations that discriminate between different, single-component, crystal structures have been identified [1] via trial and error, their binary counterparts do not yet exist. Here we focus on binary mixtures of hard spheres of various size ratios for which several crystal structures are predicted
to be stable. We develop a supervised artificial neural network to function as an order parameter that ‘learns’ to distinguish different binary crystalline and fluid environments on a single particle basis,
by analyzing vectors composed of several averaged local bond order parameters.
The training dataset for the network is produced by performing Monte Carlo (MC) simulations of the crystals under study. Once the network is trained, the classification process is computationally very cheap, so that it can easily be employed in real-time during simulations. Figure 1 shows the classification of hard spheres in a simulation of coexistence between one of the Laves phases, MgCu2, and an FCC crystal of large particles.
Figure 1: Neural network classification of a configuration of hard spheres displaying a coexistence of MgCu2 (brown) and FCC (orange) crystals . Blue particles represent the fluid, and are located at the disordered interface between the crystalline regions.
[1] W. Lechner and C. Dellago, J. Chem. Phys. 129, 114707 (2008)